Our research group works in the field of Uncertainty Quantification and Inverse Problems. Our team, at the School of Engineering Sciences, is part of the Centre of Excellence Inverse Modelling and Imaging (2018-2025), and of the Flagship of Advanced Mathematics for Sensing, Imaging and Modelling (2024-2031), both granted by the Research Council of Finland. Other partners of the consortia are University of Helsinki, Aalto University, University of Eastern Finland, University of Jyväskylä, University of Oulu, Tampere University, and Finnish Meteorological Institute.
Inverse problems appear in several fields, including medical imaging, image processing, mathematical finance, astronomy, geophysics, nondestructive material testing and sub-surface prospecting. Typical inverse problems arise from asking simple questions "backwards". For instance, the simple question might be "If we know precisely the structure of the inner organs of a patient, what kind of X-ray images would we get from her?" The same question backwards is "Given a set of X-ray images of a patient, what is the three-dimensional structure of her inner organs?" This is the inverse problem of Computerized Tomography, or CT imaging.
Usually the inverse problem is more difficult than the simple question that it reverses. Successful solution of inverse problems requires specially designed algorithms that can tolerate errors in measured data. Our research group focuses on uncertainty quantification in inverse problems. We specialise in the specific field of Bayesian statistical inverse problems, which is the effort to address real-world inverse problems as Bayesian statistical estimation problems. We study how statistical errors in measured data propagate to solutions within the complex mathematical models of inverse problems.
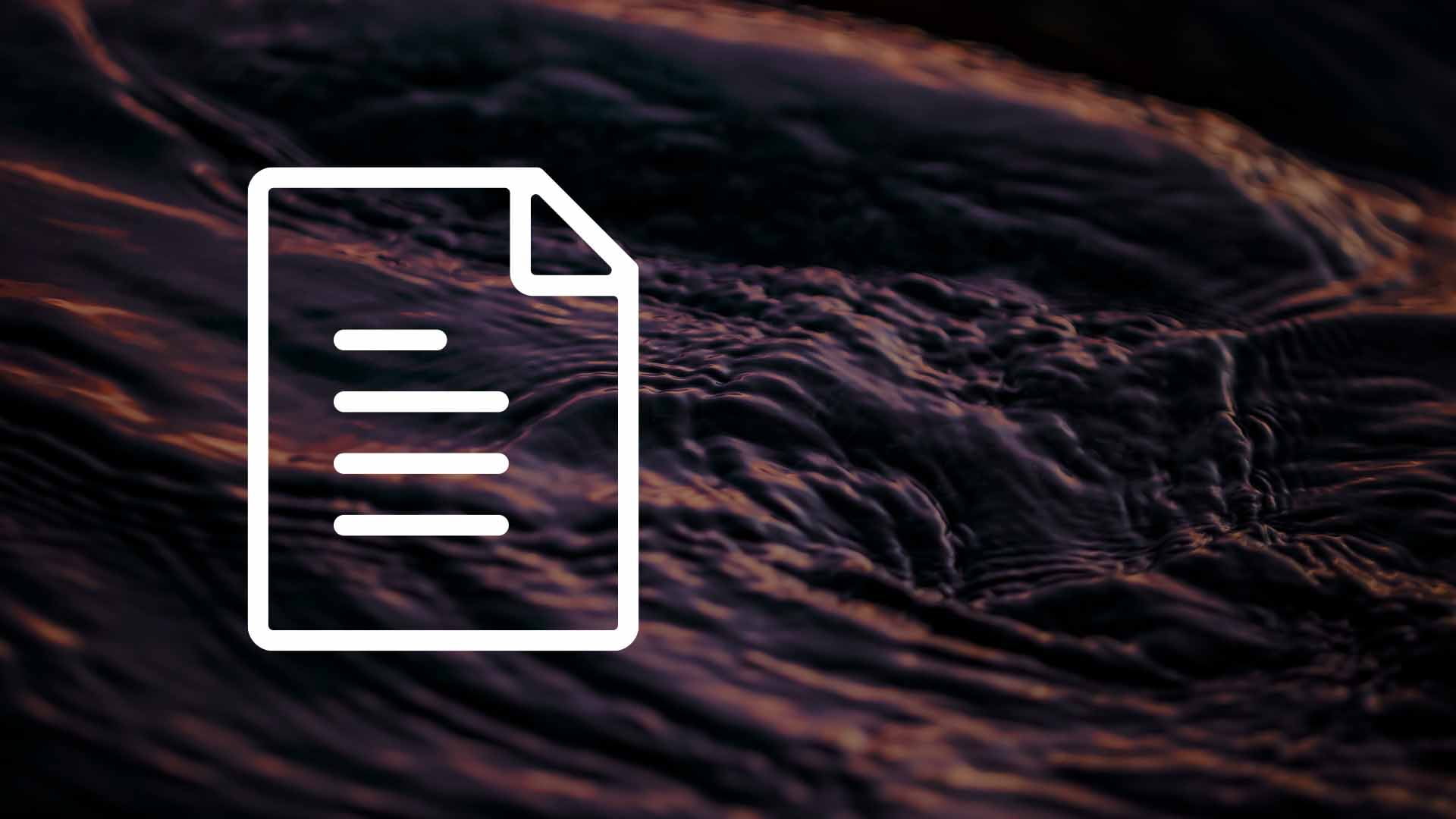
Seminar on Computational Engineering
The Seminar on Computational Engineering at the LUT University is usually held in room 2411 on Tuesdays at 2pm. Our aim is to bring together computational science with applied mathematics and statistics.
Mathematical Foundations
Bayesian statistics recasts the inverse problem in the form of statistical quest of information. The degree of belief in different solution candidates are described by prior probabilities. Using the Bayes' theorem this information is updated to posterior probabilities based on the measurement data. Due to high dimensionality typical to inverse problems, exploring the uncertainty of the solutions require exceptionally large computational effort. Fundamental theoretical and numerical development is crucial to make uncertainty quantification feasible for the massive data sets becoming available in applications. Our team develops theory, numerical algorithms and practical implementations related to uncertainty quantification and inverse problems, a highly interdisciplinary field of science between applied mathematics and statistics. We have been forerunners of Adaptive MCMC algorithms, a class of more effective MCMC algorithms, now widely used in various applications. Adaptive MCMC methods learn from the previous model evaluations ‘on fly' and tune the algorithm as the simulation proceeds. A Toolbox with more details is available at GitHub.
Large-scale Research Infrastructure Projects
We are involved in several large-scale research infrastructure projects, the PIs and examples include:
Prof. Heikki Haario collaborates with FMI (the Finnish Meteorological Institute) on weather and climate models, as well as on many satellite remote sensing projects, like ENVISAT/GOMOS. At the moment one of the hottest topics is the OCO-2 satellite (Orbiting Carbon Observatory-2). The group concentrates on mathematical methodology for enabling parameter estimation in collaboration with e.g. MIT and JPL/Caltech (see the FMI blog of Janne Hakkarainen).
Prof. Tapio Helin is involved with European Southern Observatory, where atmospheric turbulence is a major challenge in modern ground-based telescope imaging as the turbulence (fluctuations of the refractive index) perturbs the light waves passing through atmosphere. In telescope imaging this phenomenon causes degraded resolution and blurring of the images. A good mathematical model of the atmospheric turbulence is essential in estimating how accurate imaging is possible at given weather conditions. Our research takes a reversed perspective. Consider a light wave entering the atmosphere and being observed on the ground. What information can such data provide from turbulence in the atmosphere? This inverse problem becomes a crucial challenge in the ground-based telescope imaging during the next decade.
Prof. Lassi Roininen is involved with building the next-generation 30,000-antenna fully-digital phased-array high-power incoherent scatter radar EISCAT3D. With transmitter-receiver in Norway and receivers in Finland and Sweden, and radar first light 2021, it will provide 3D volumetric radar imaging of the ionospheric plasma at altitudes 80-1,000 km. EISCAT3D can be used for space weather monitoring, lunar mapping, and space debris and asteroid detection. We develop mathematically rigorous spatiotemporal volumetric radar imaging by providing powerful supercomputing solutions for large-scale computational inversion with novel Gaussian and non-Gaussian spatiotemporal hierarchical models and full-blown uncertainty quantification.
Professors
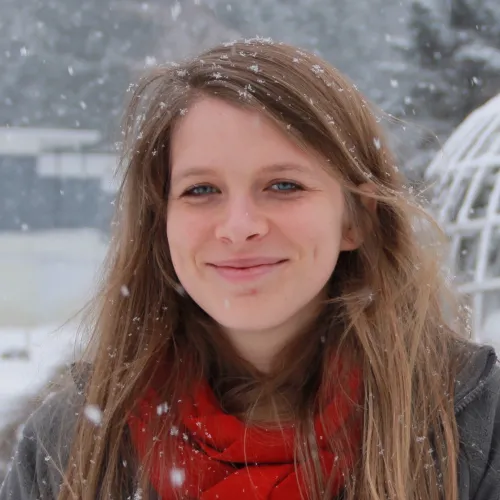
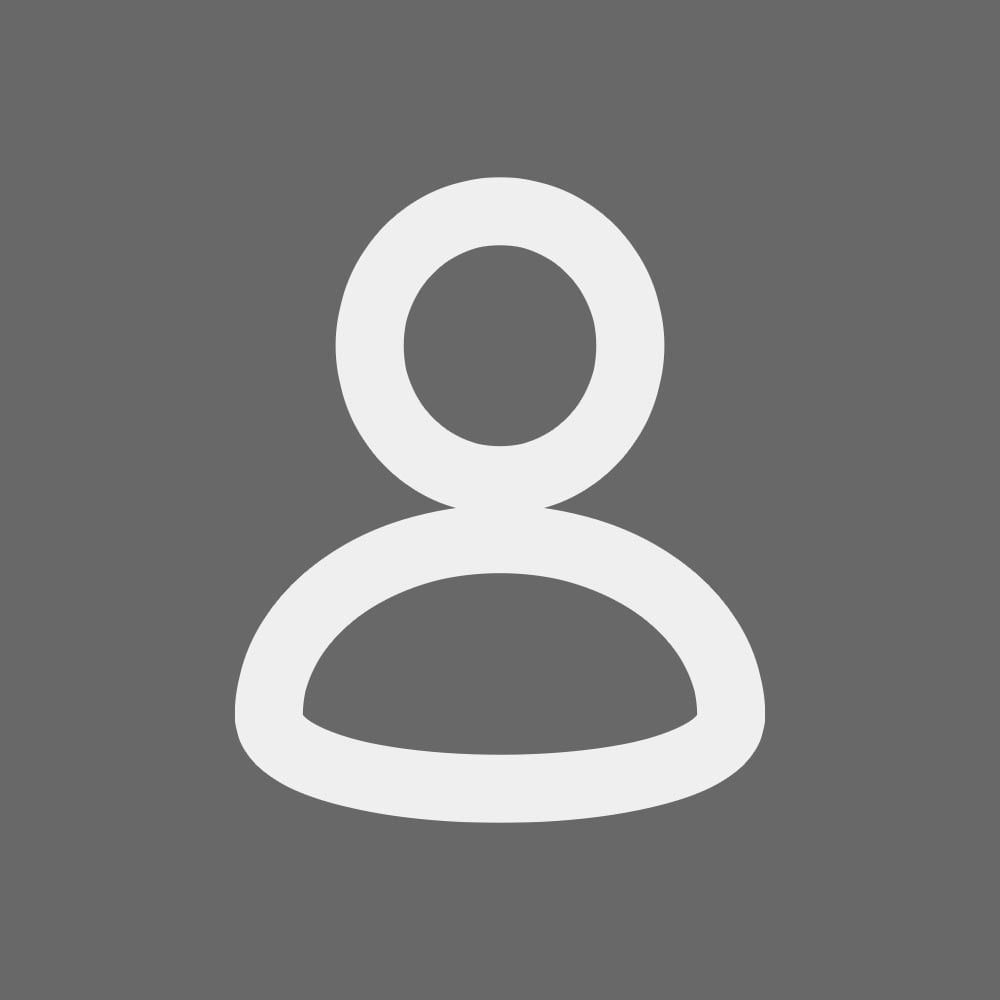
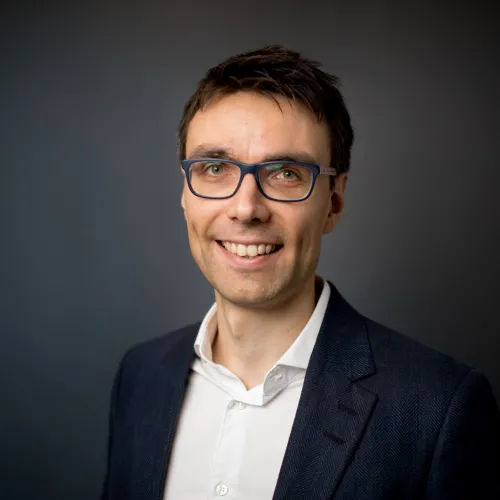
Tapio Helin
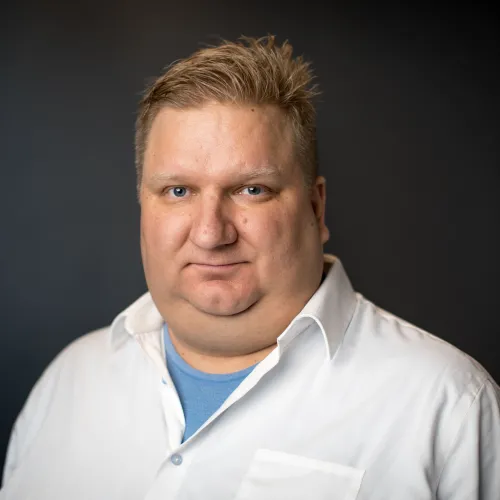
Lassi Roininen
Senior scientists
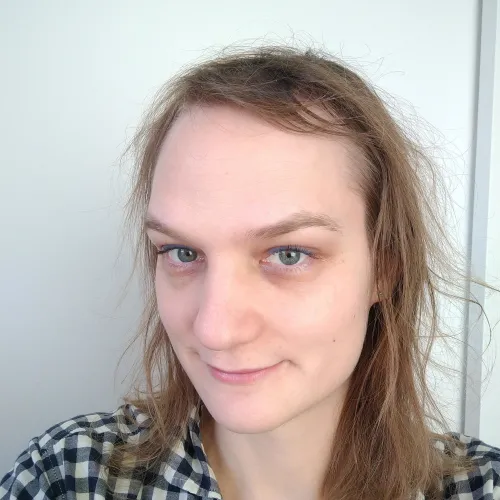
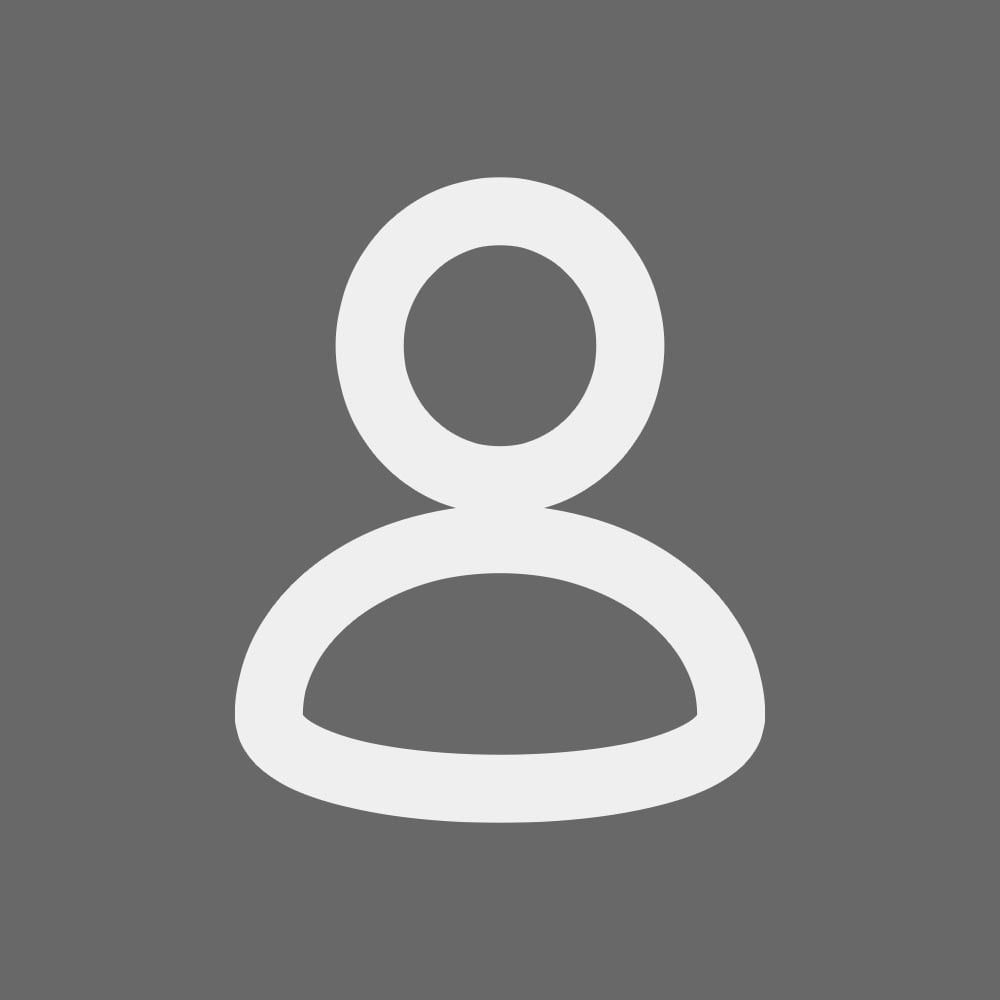
Jesse Railo
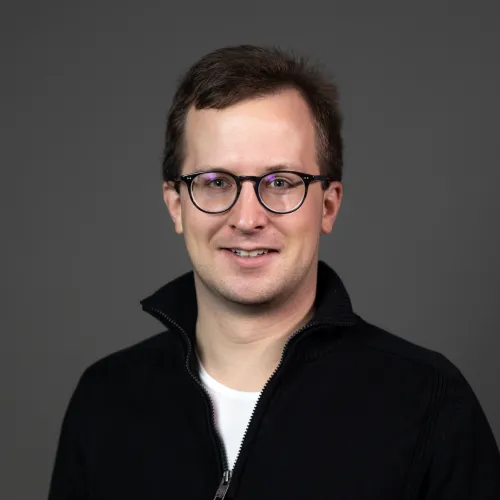
Andreas Rupp
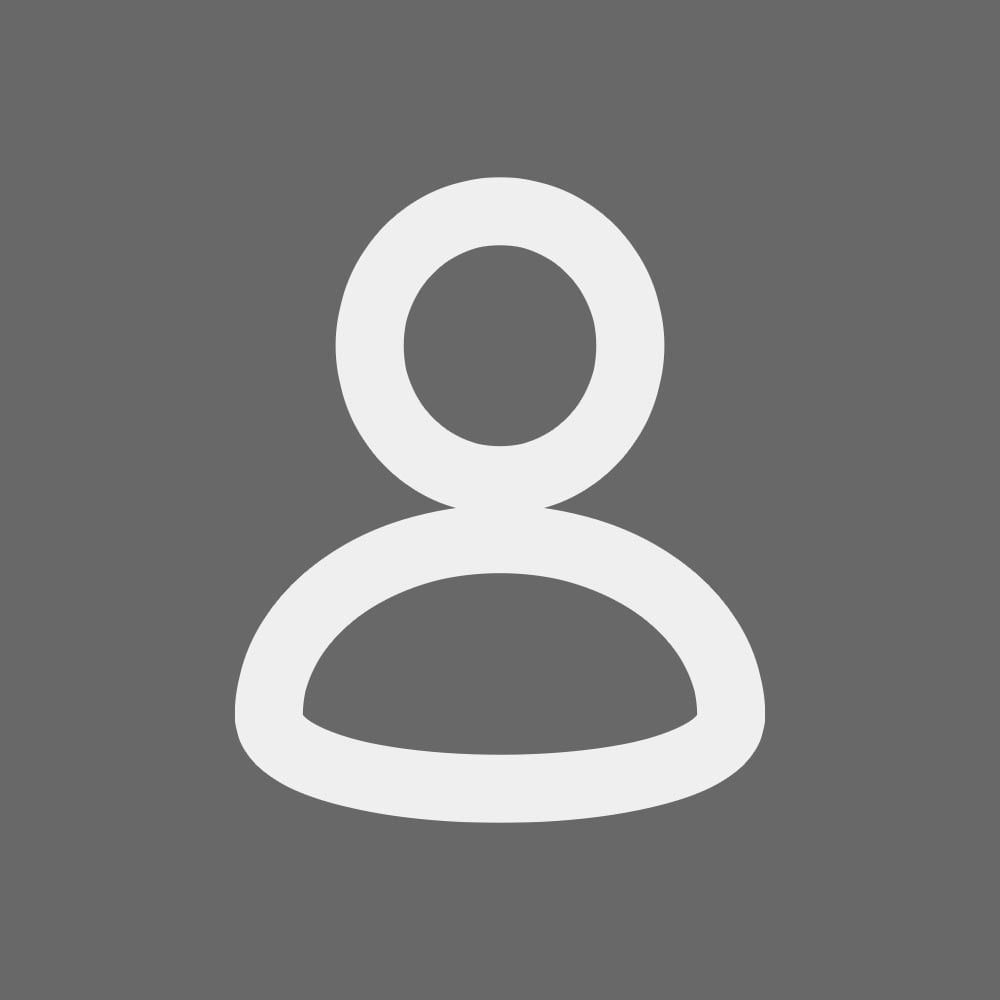
Jouni Sampo
Post-doctoral researchers
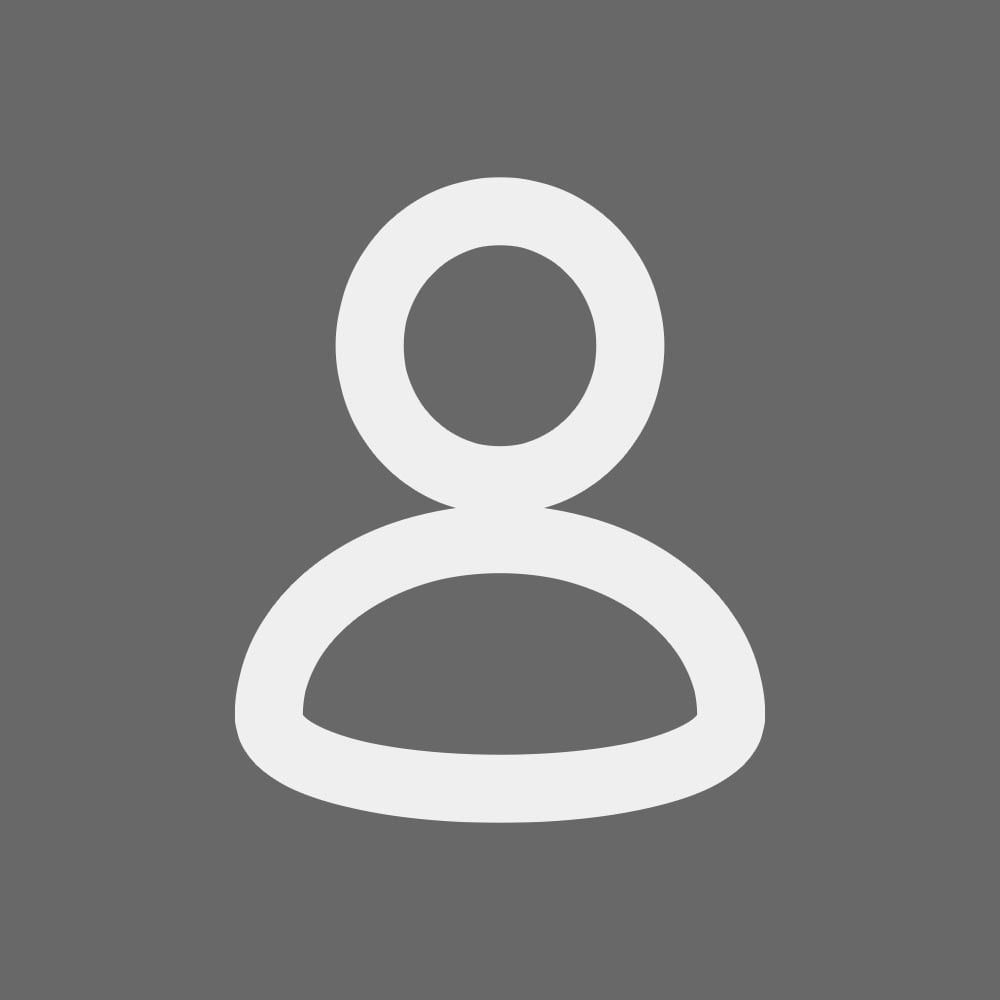
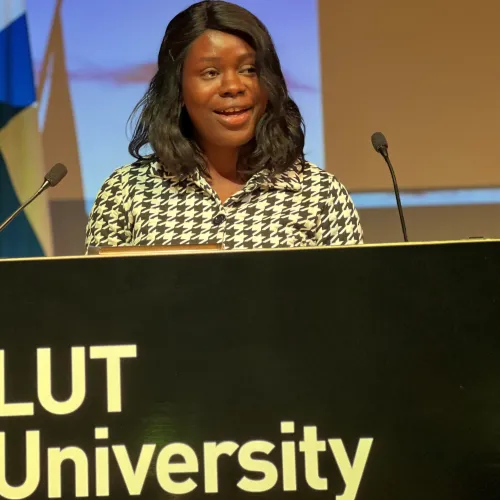
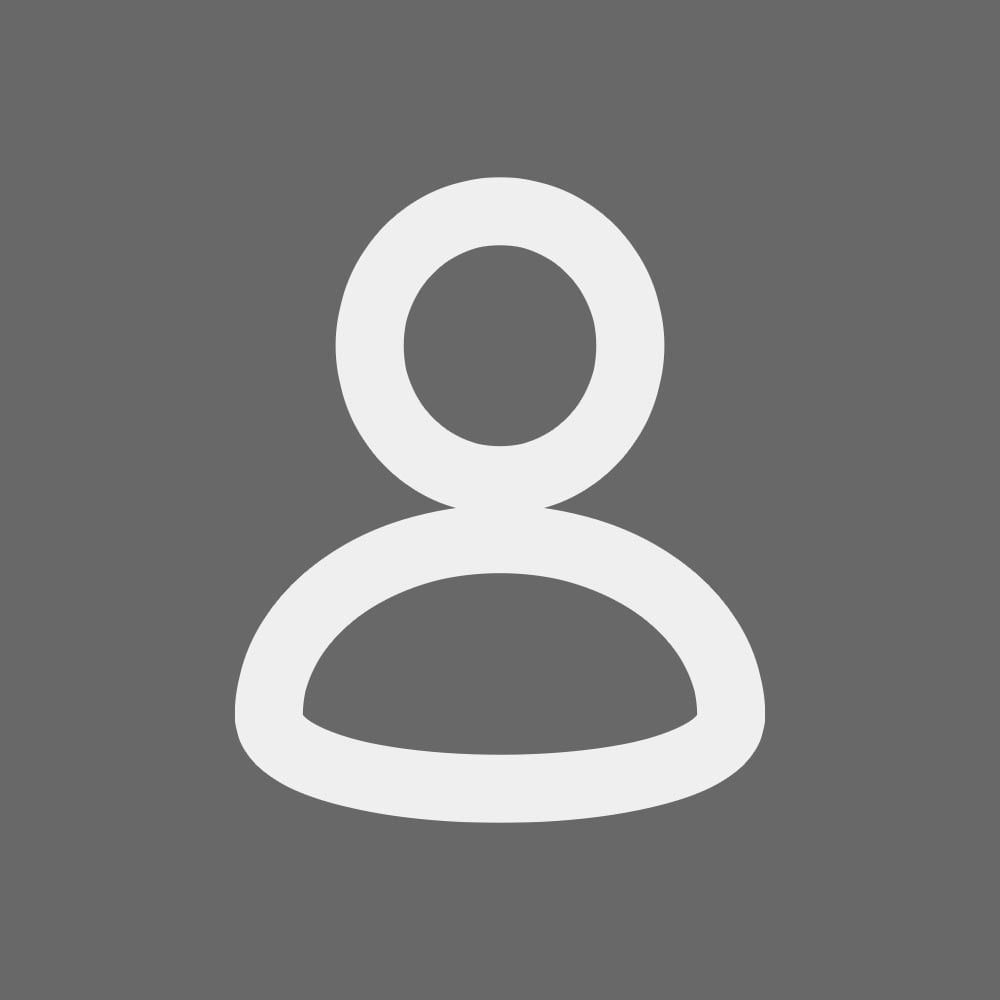
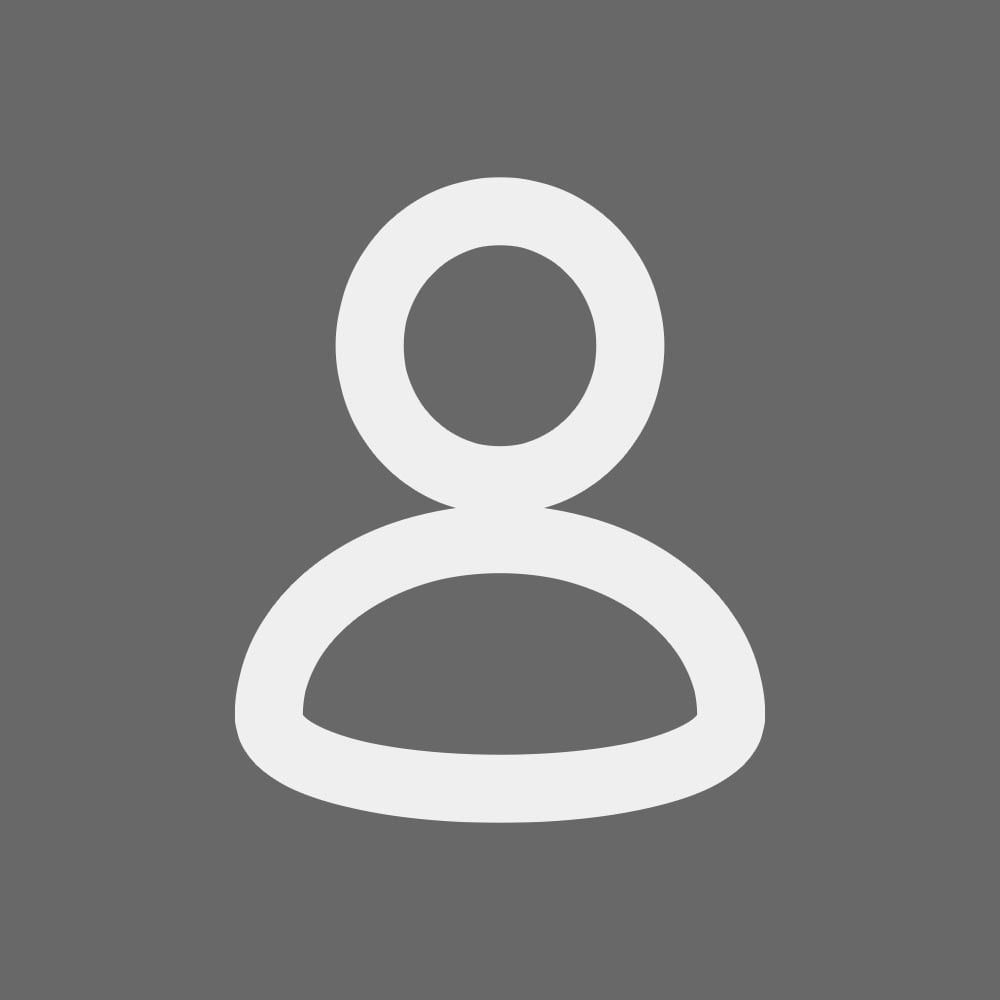
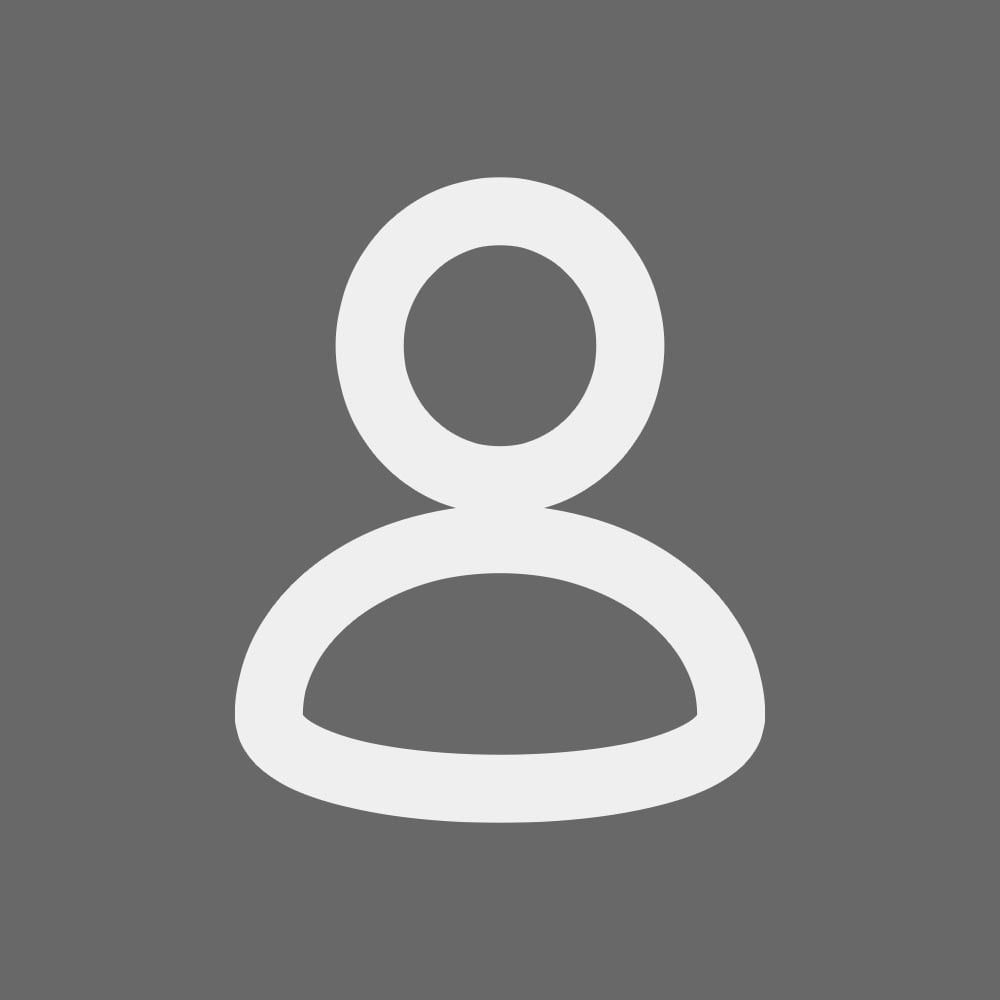
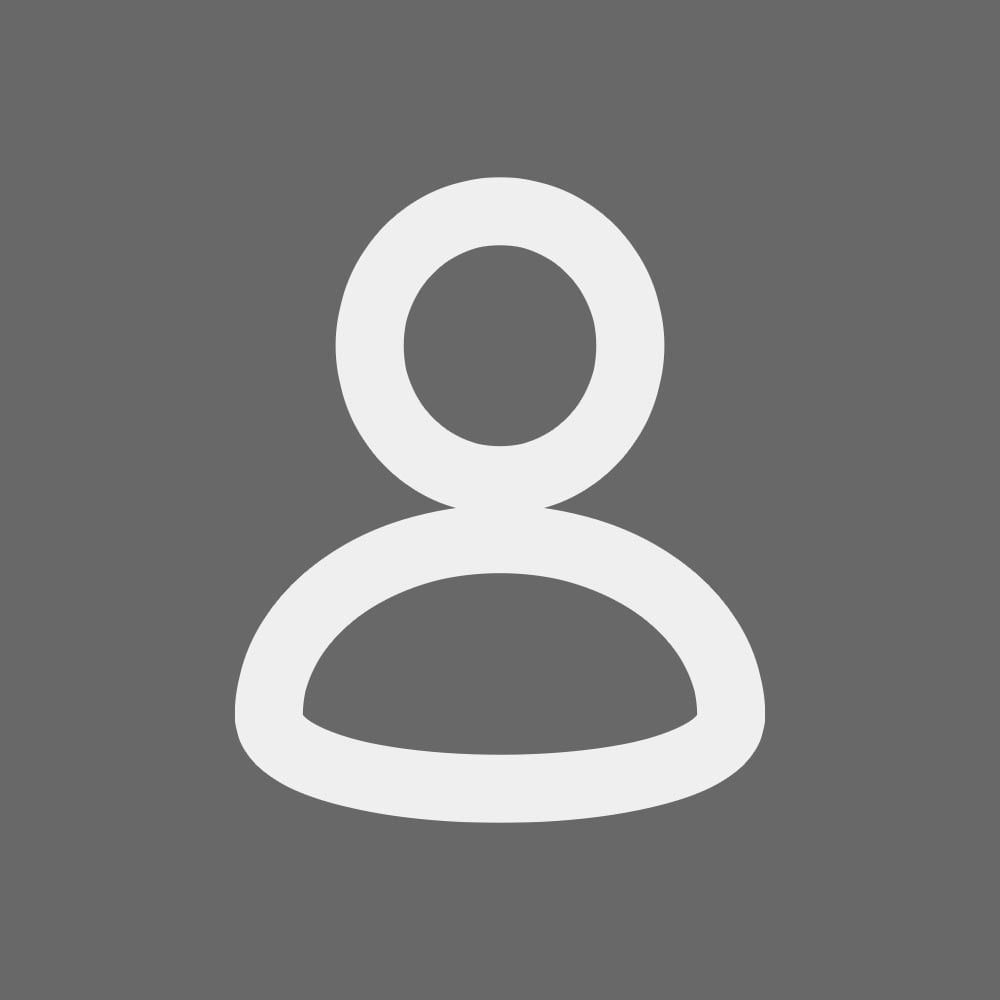
Shubham Jathar
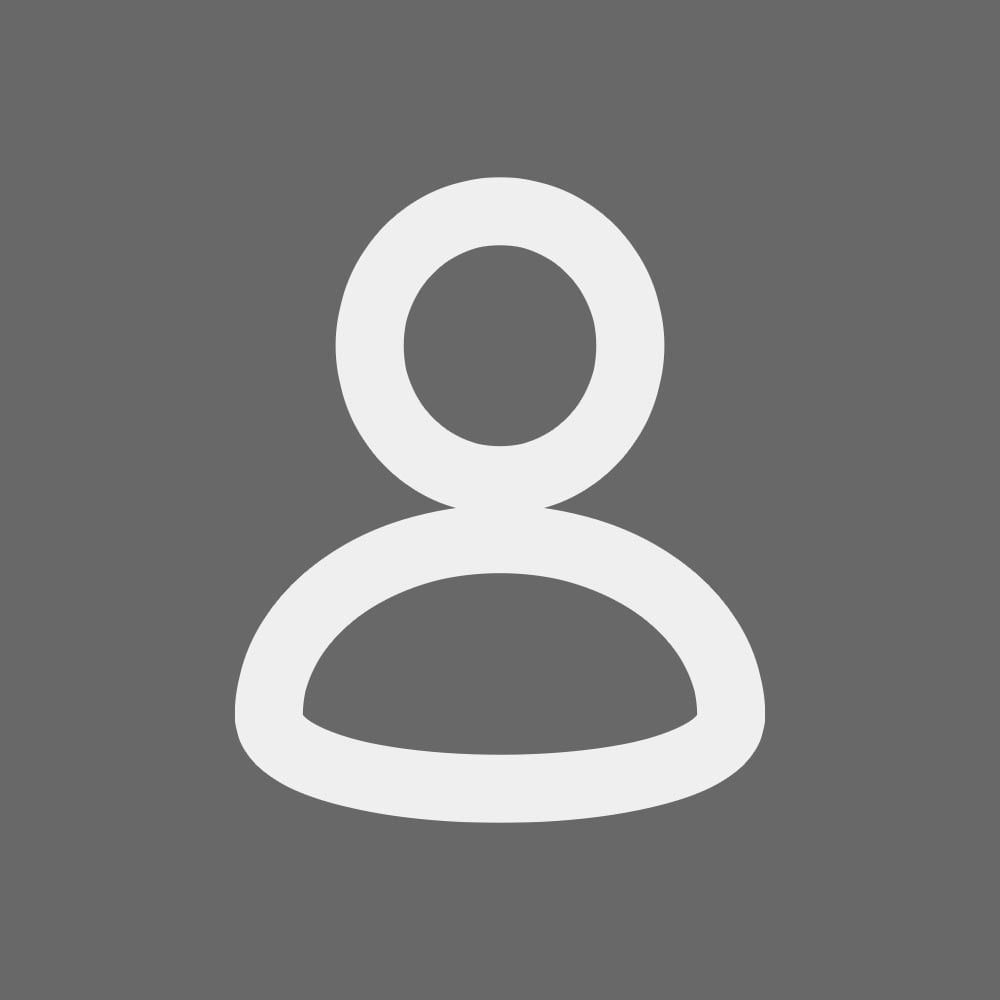
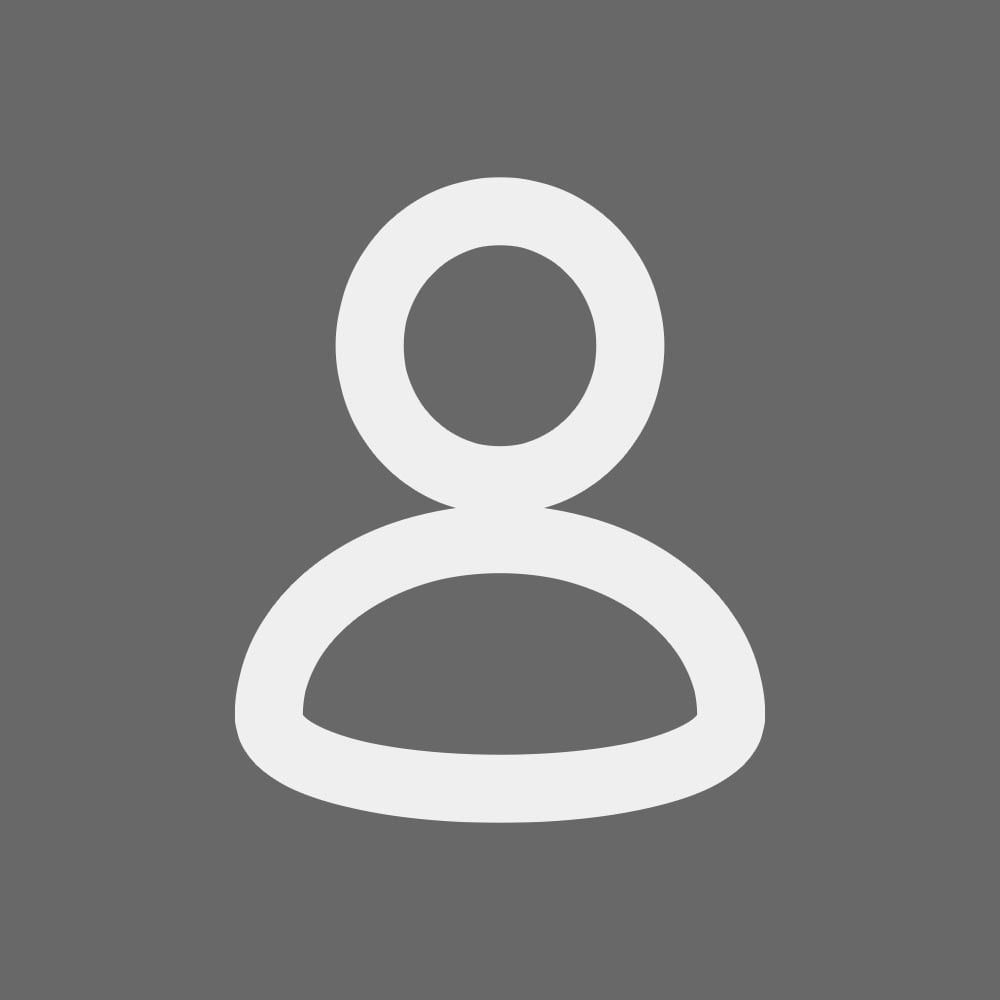
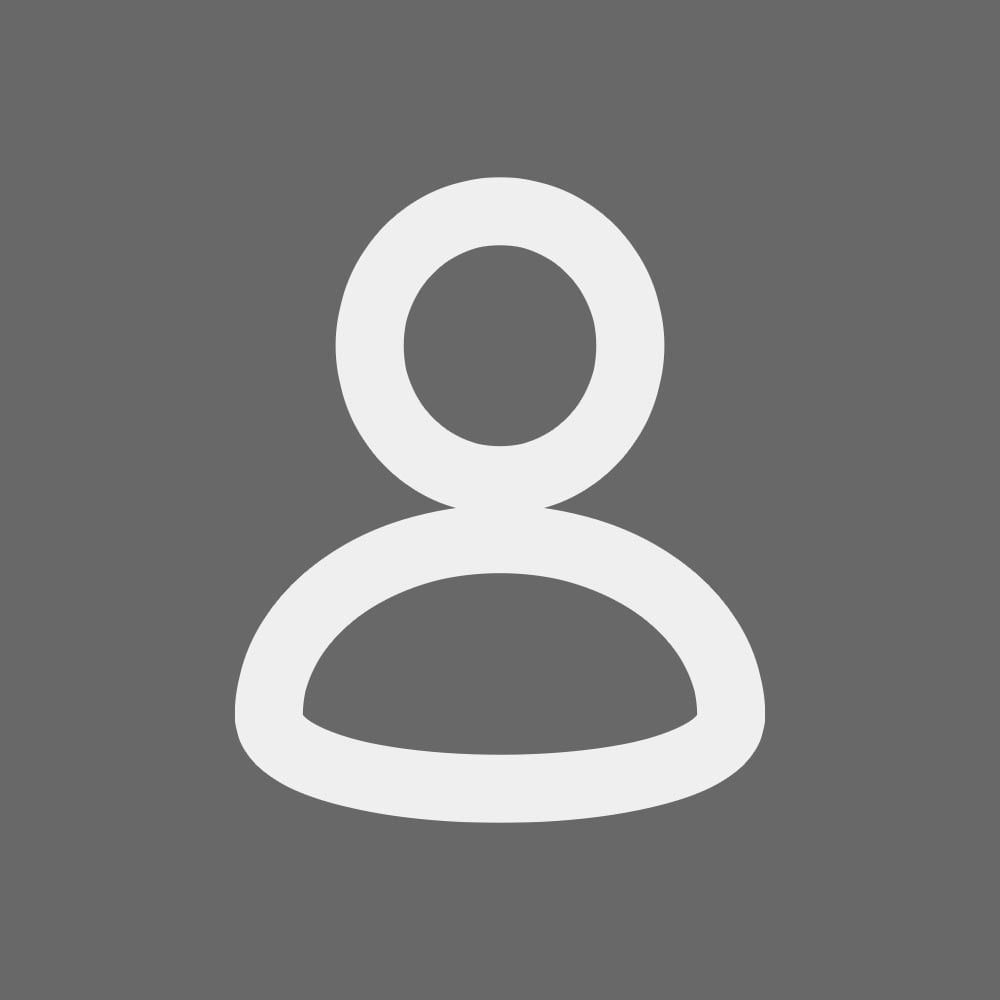
Ph.D. students
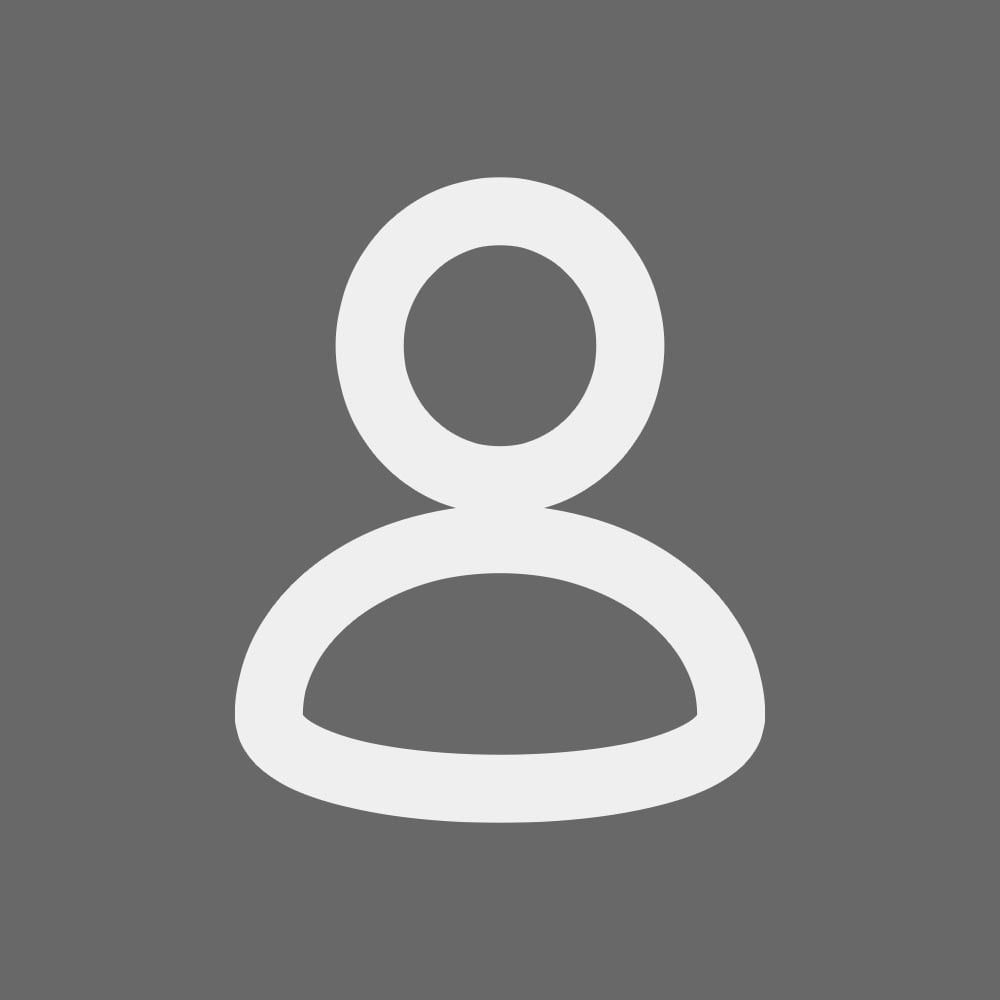
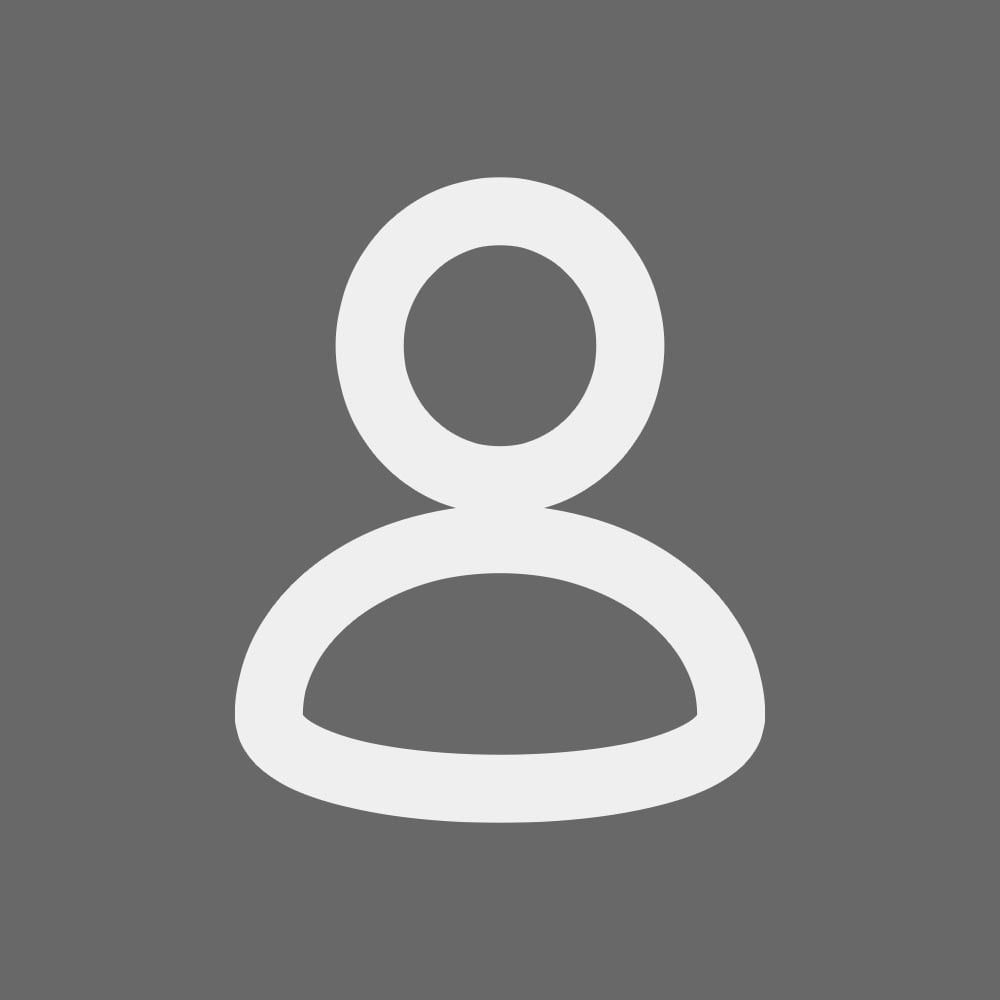
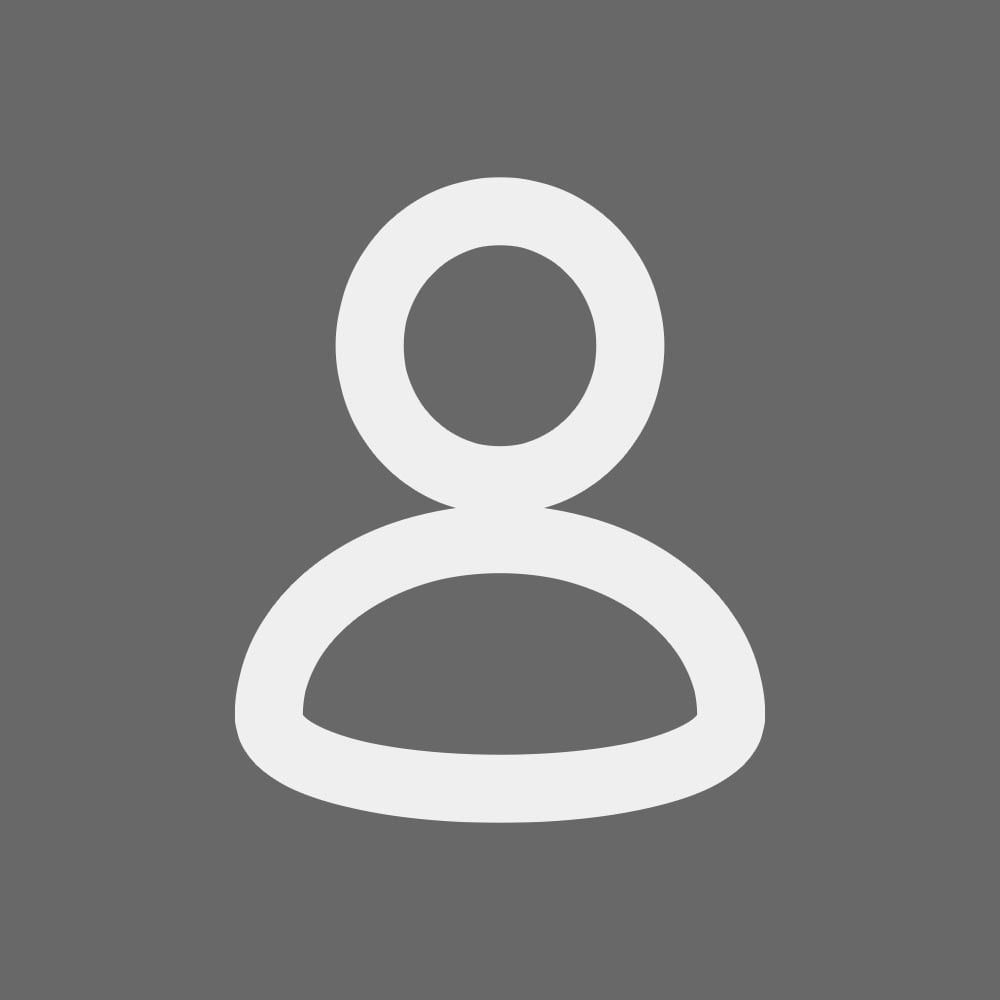
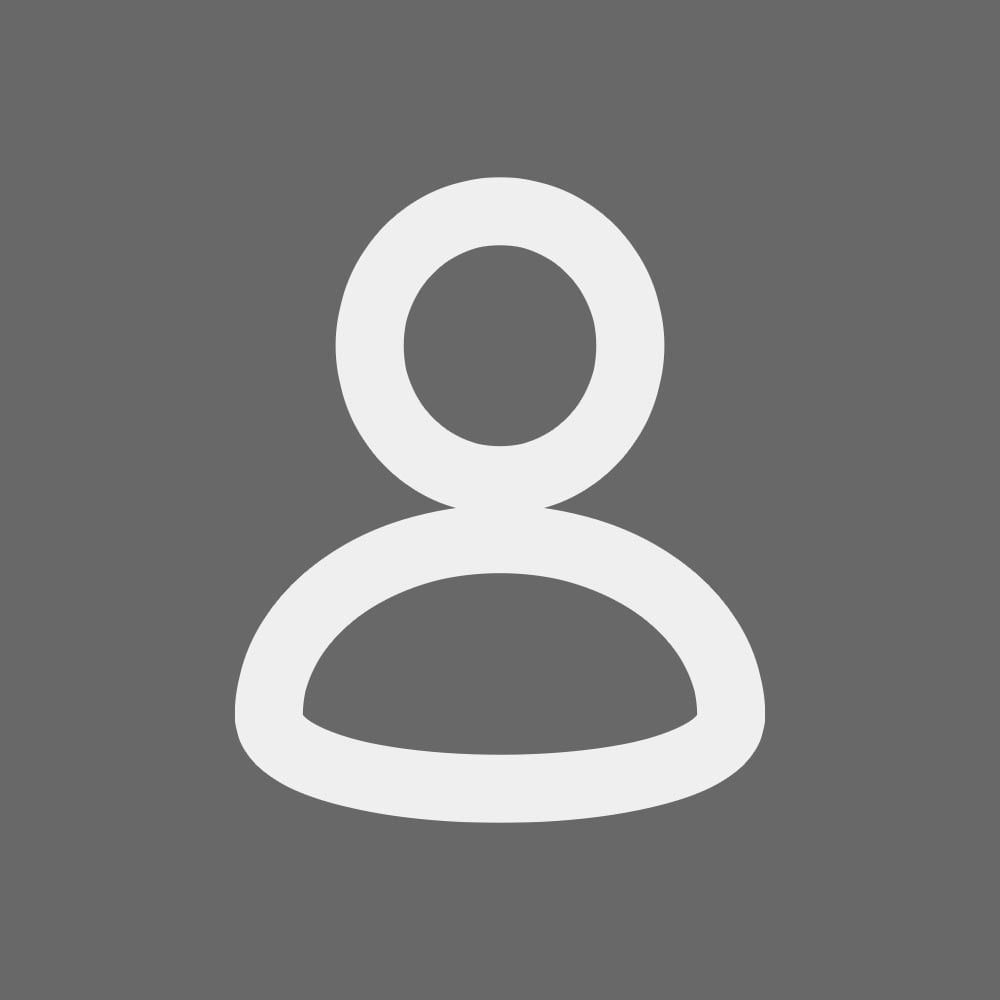
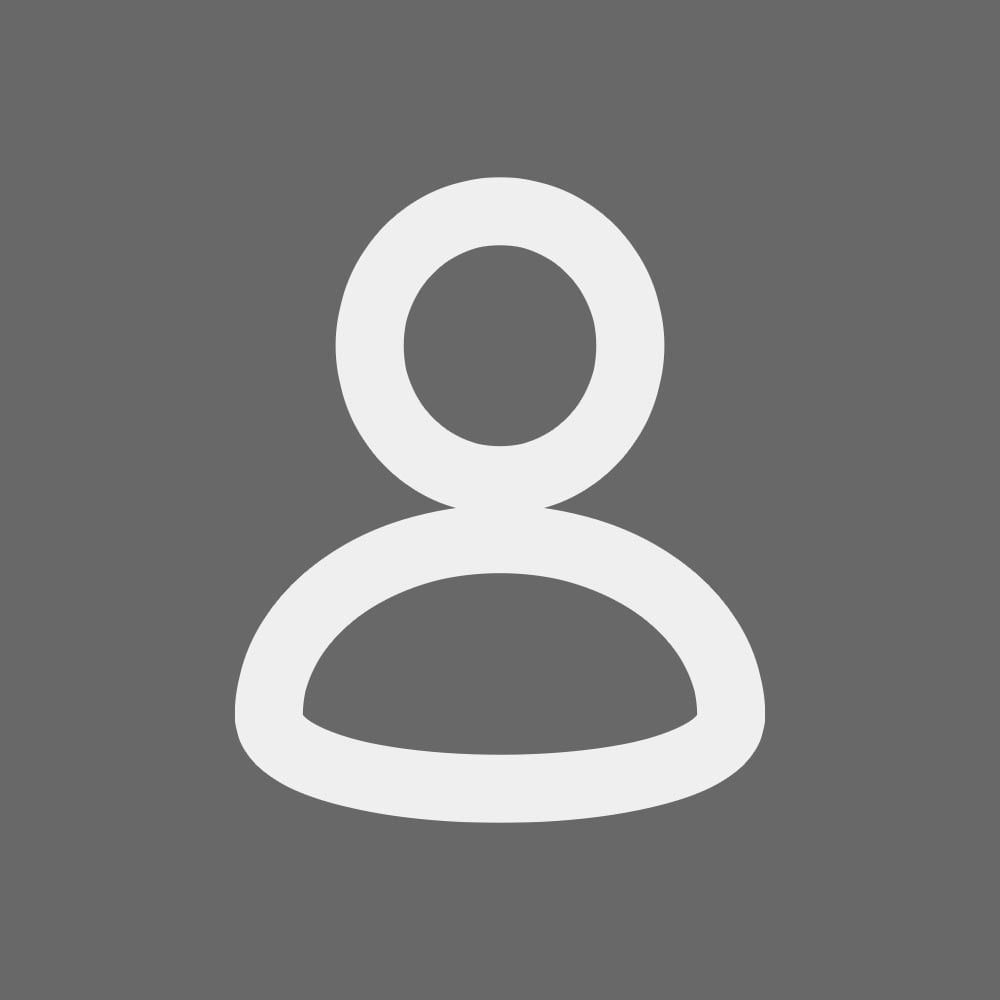
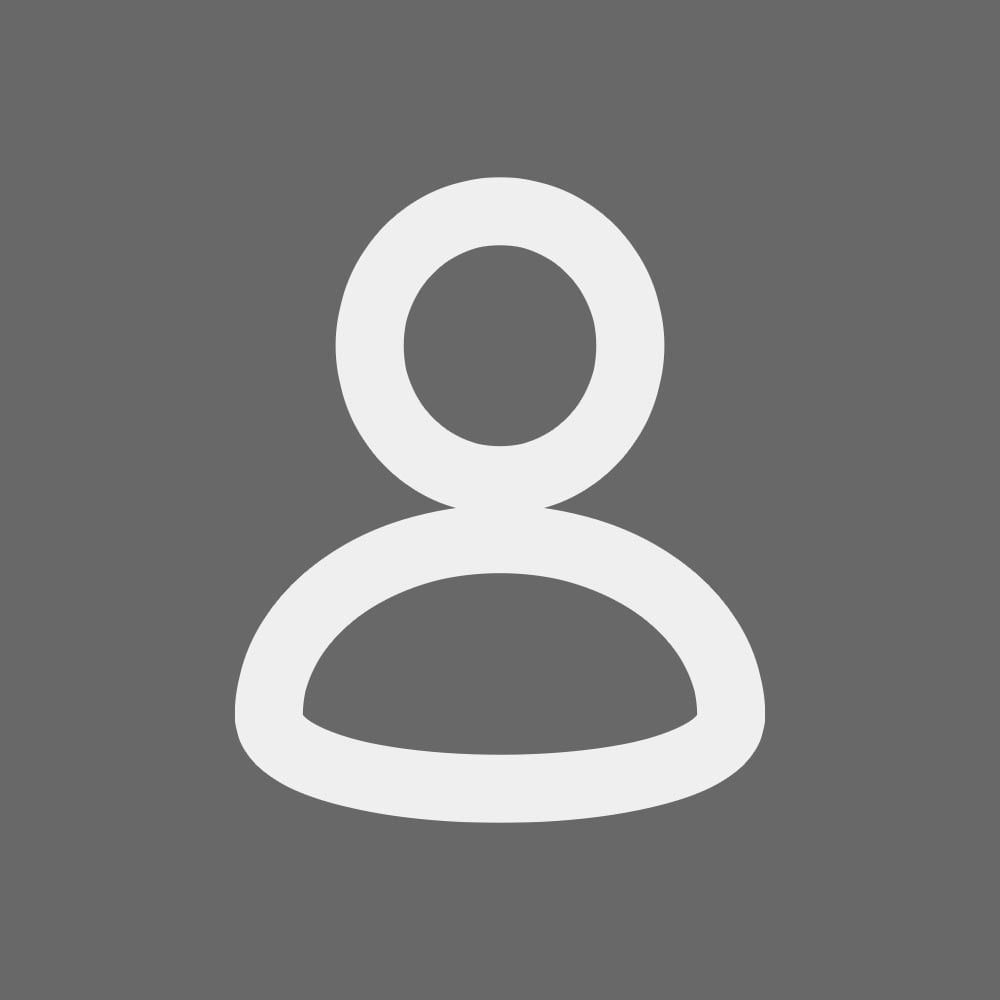
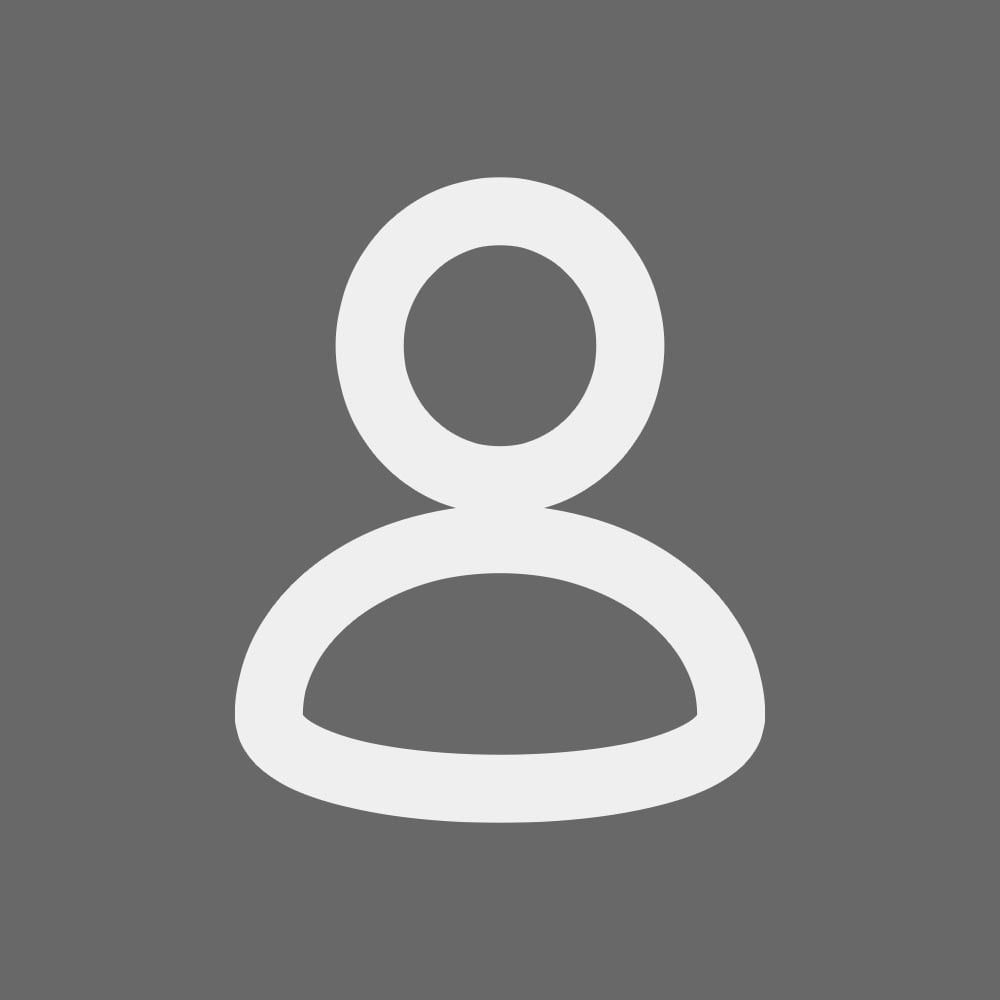
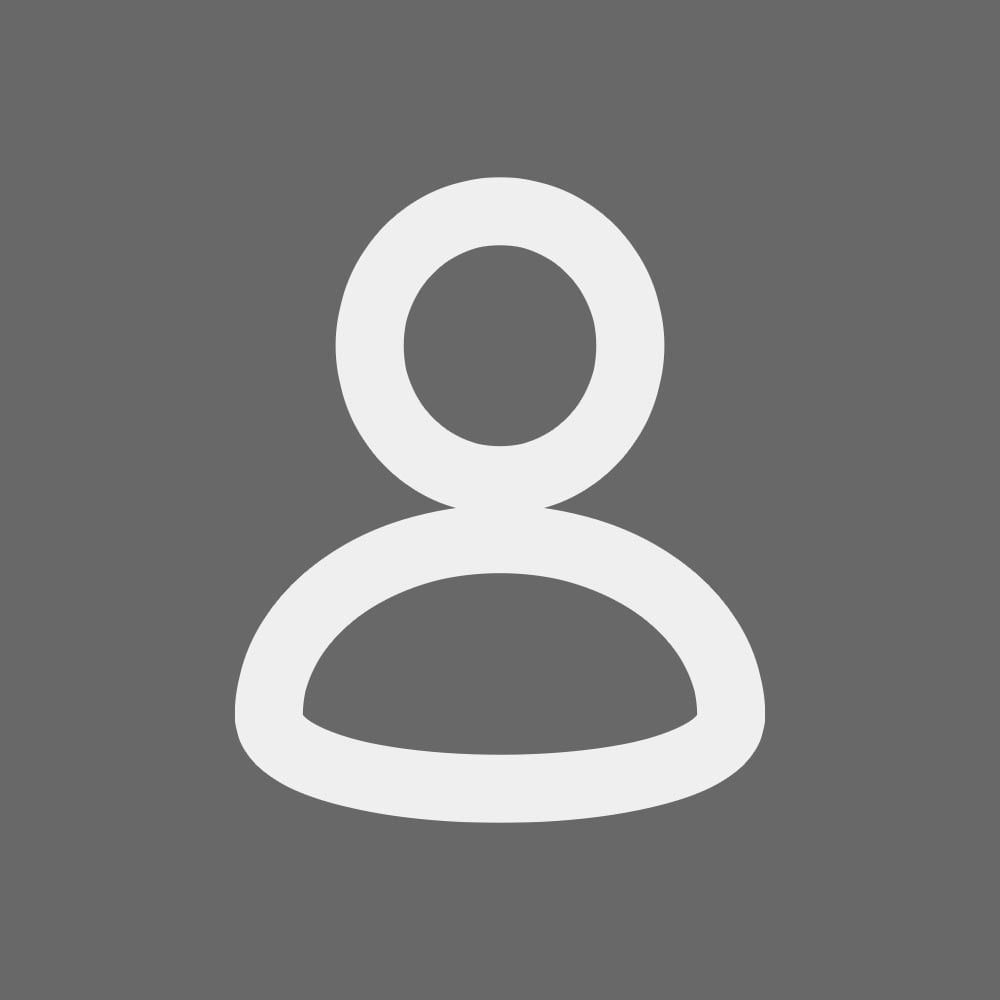
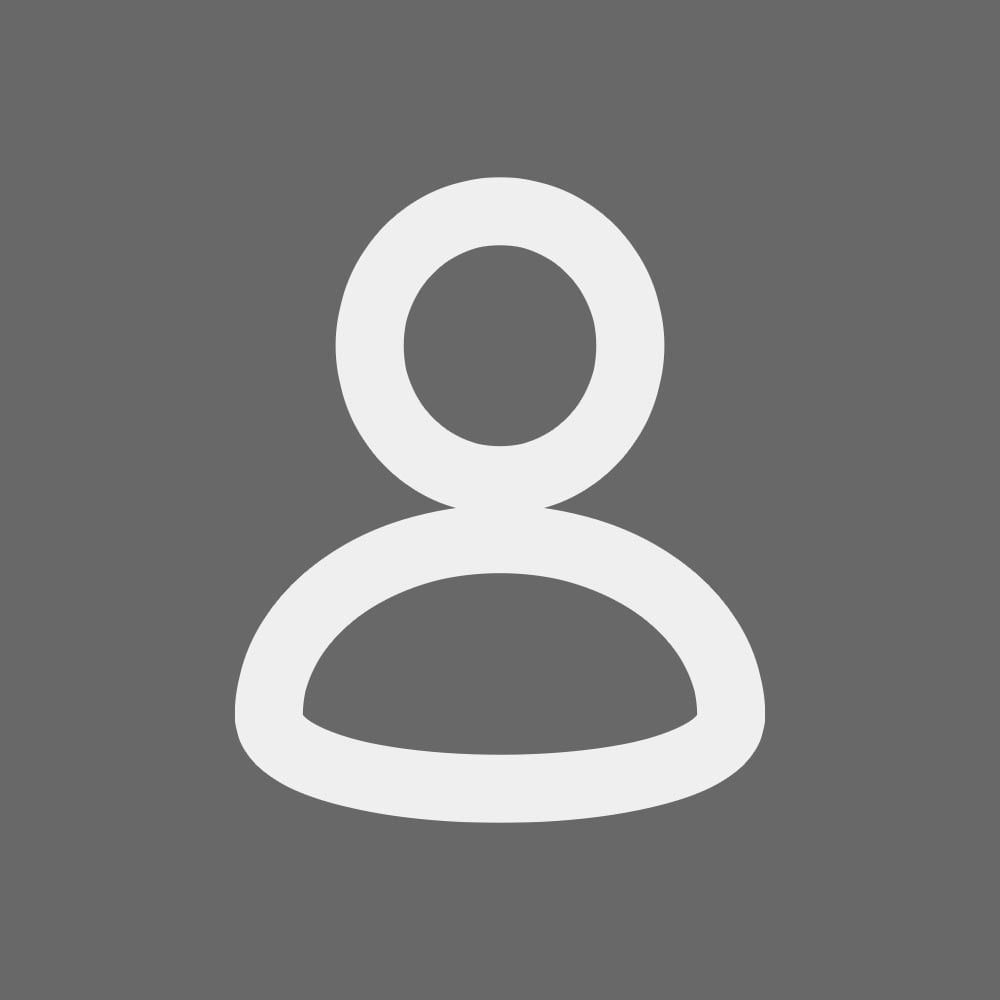
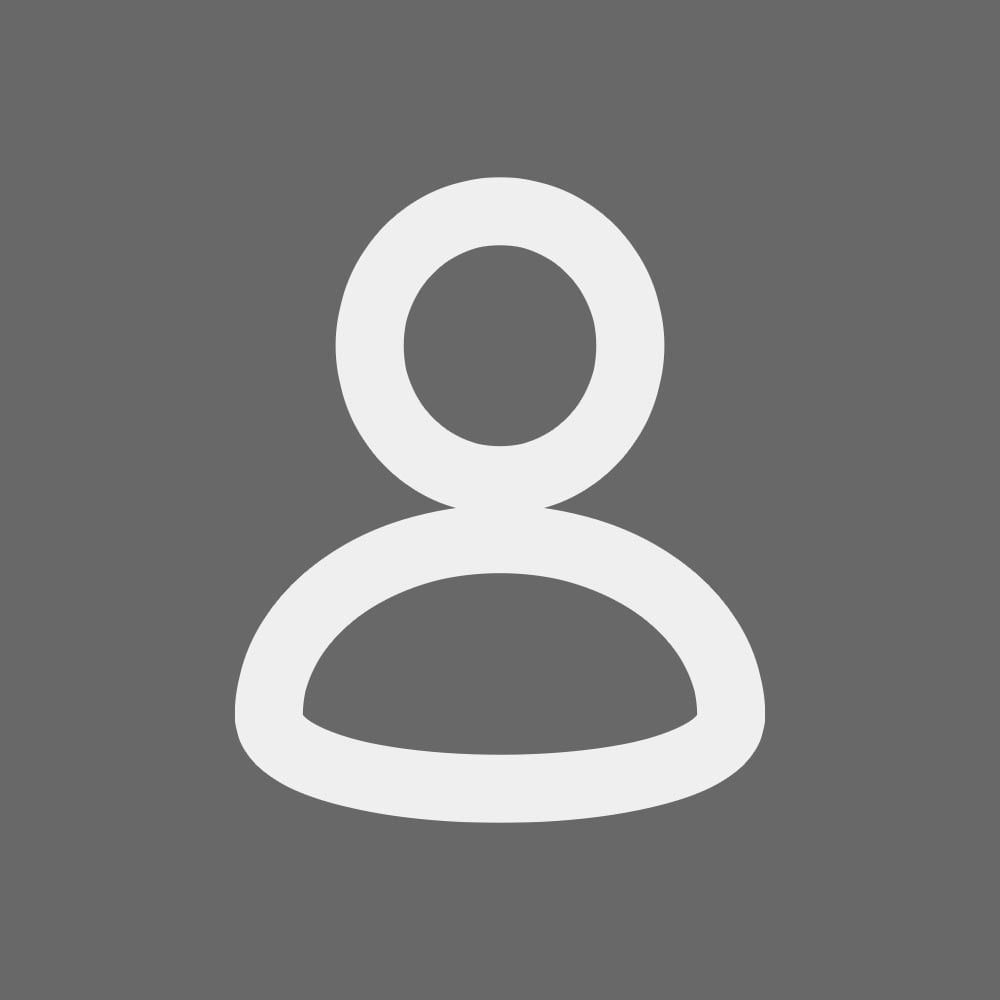
Docents
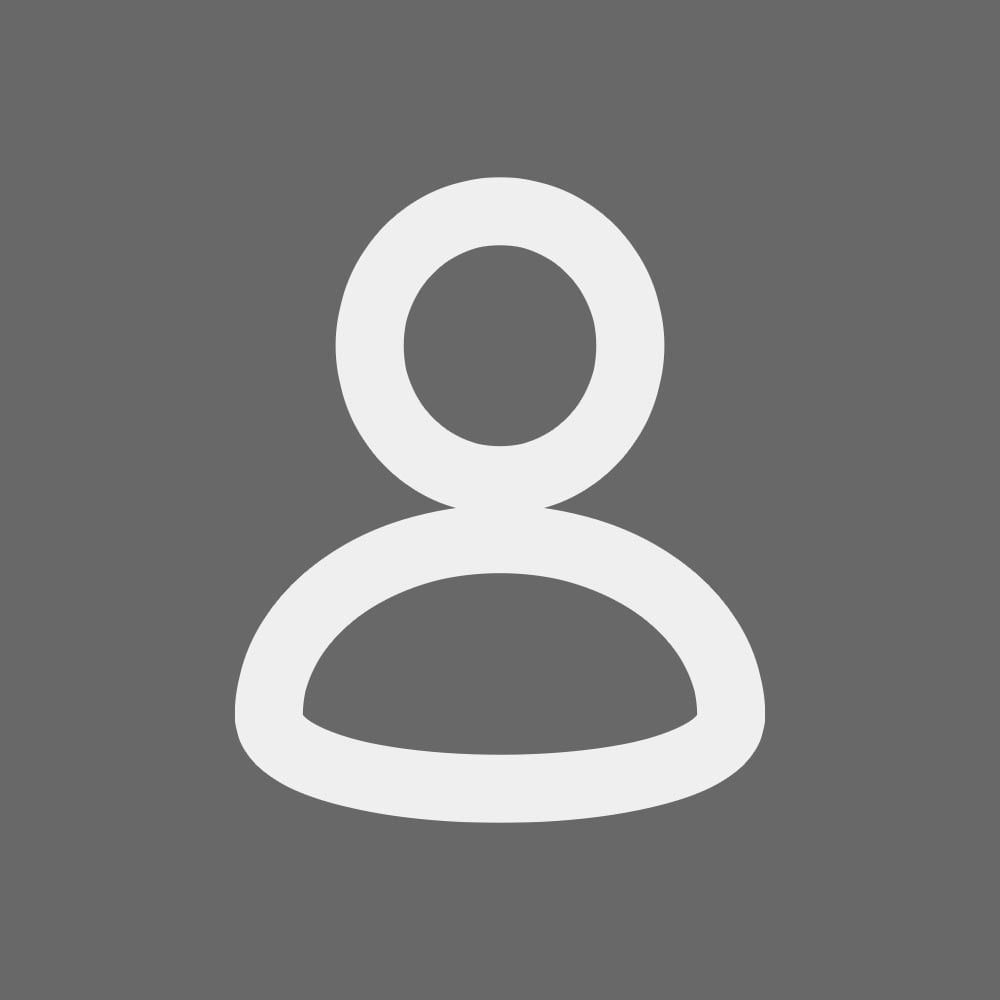
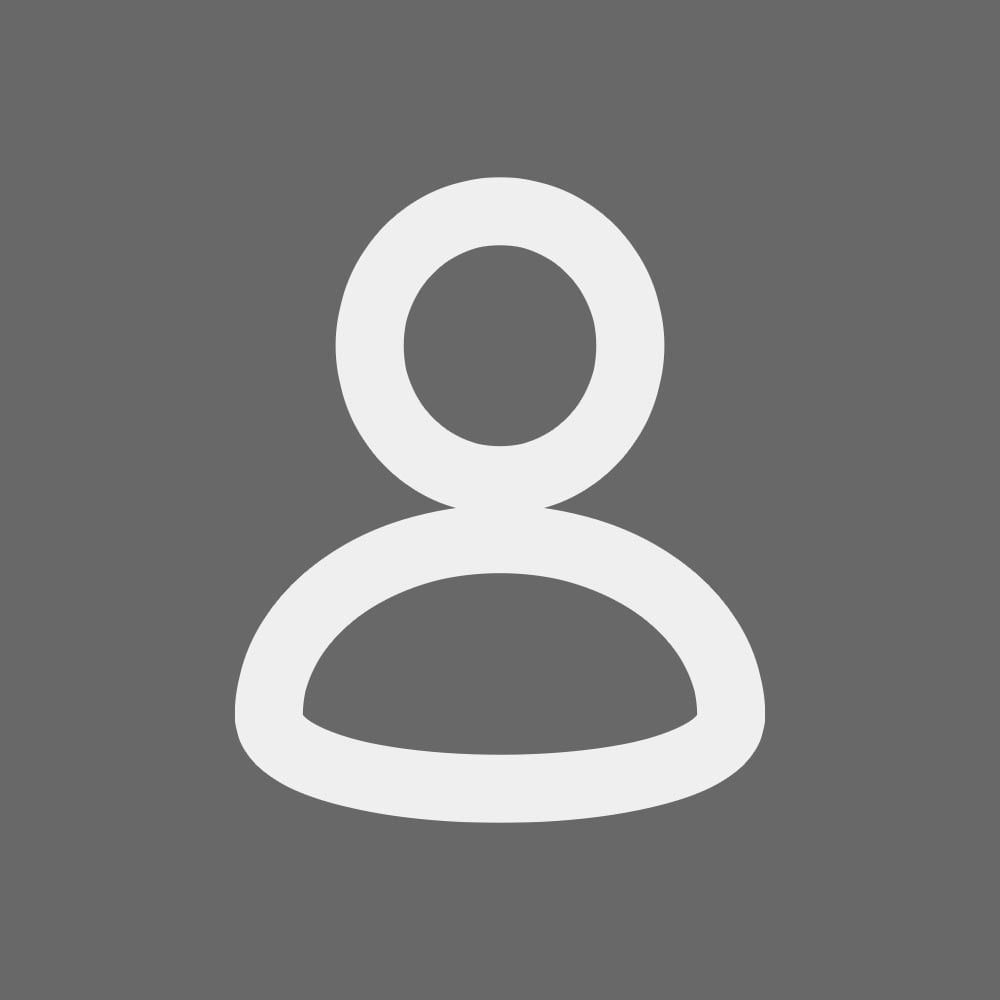
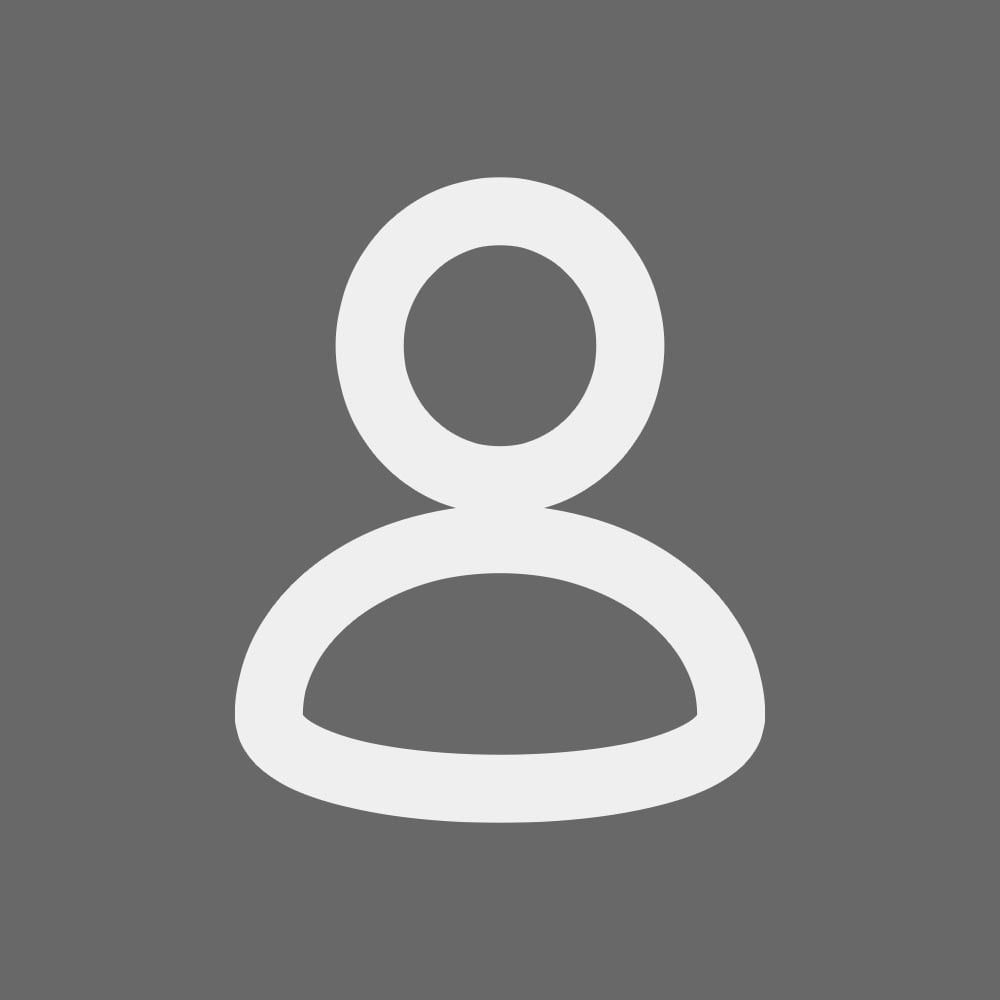
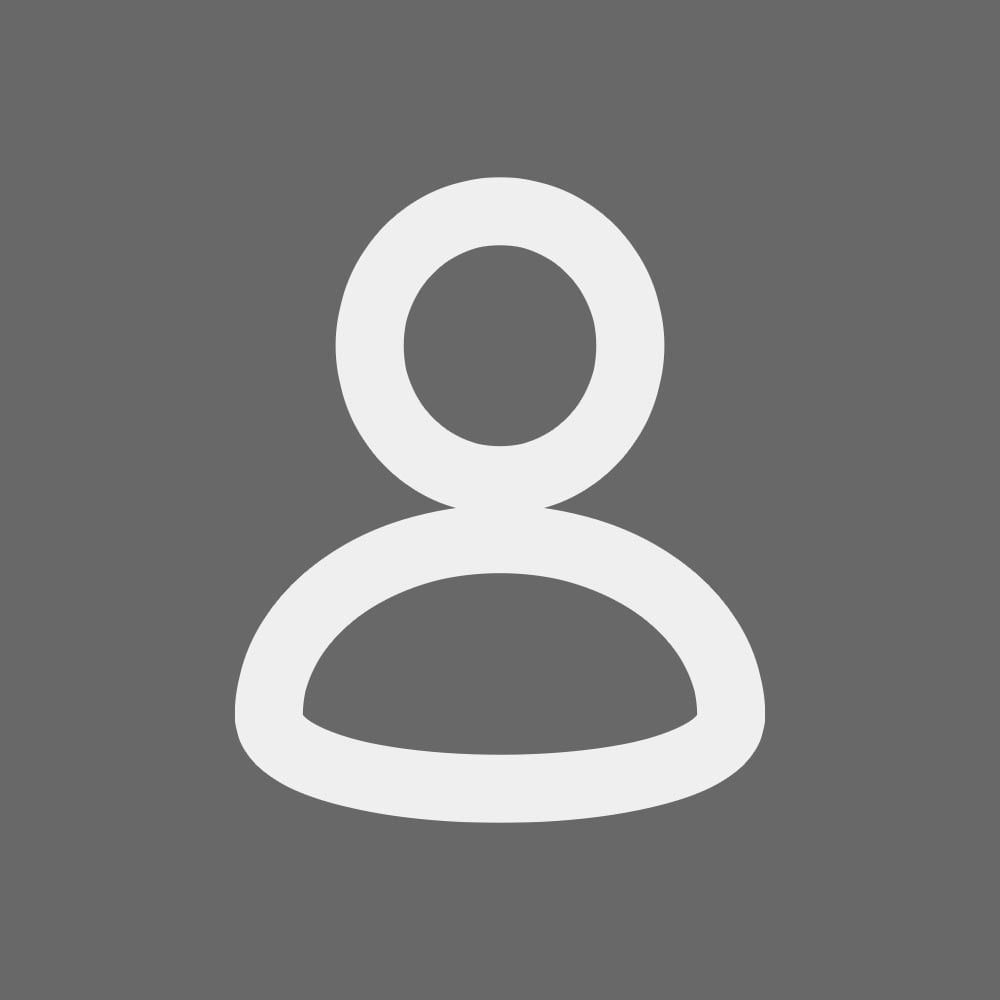
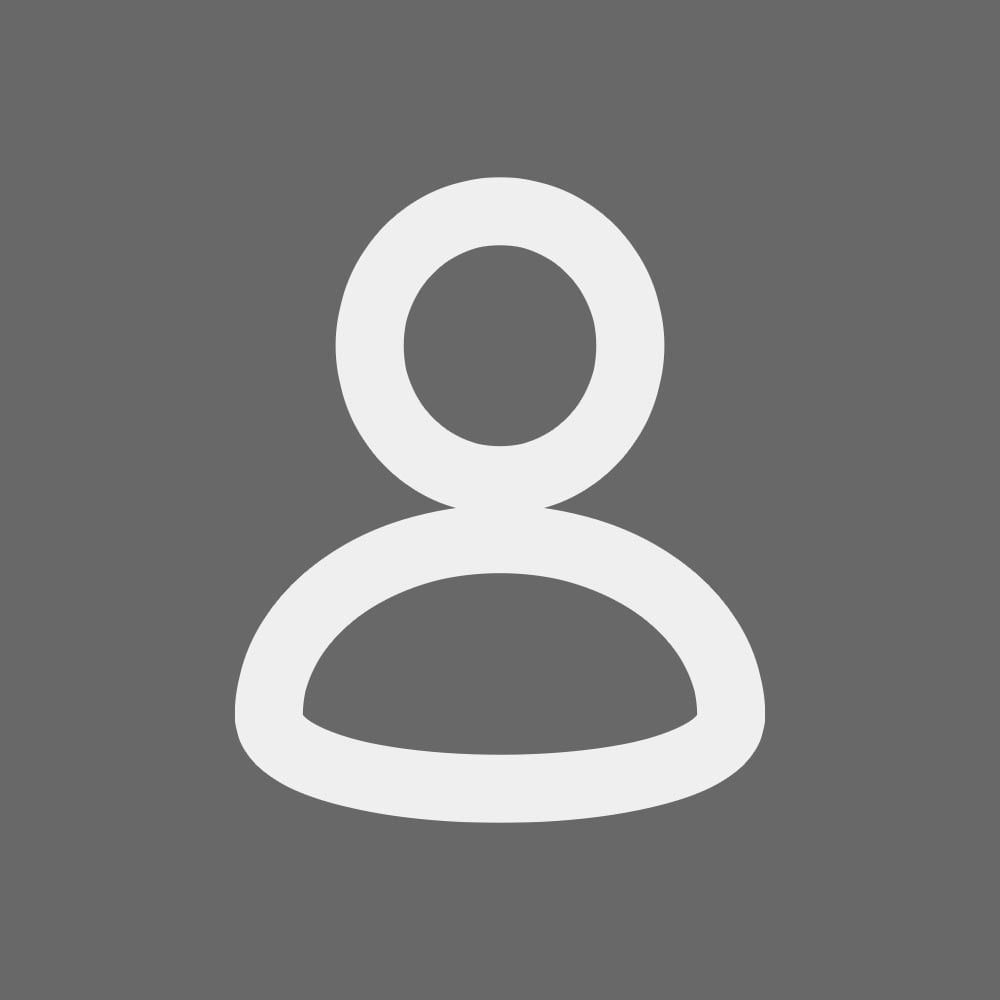
Ph.D. candidates without LUT affiliation
- Lasse Johansson
- Theonille Mukamana
- Michel Rwema